Why Is The Concept of “Human-in-the-Loop” Important When Building AI?
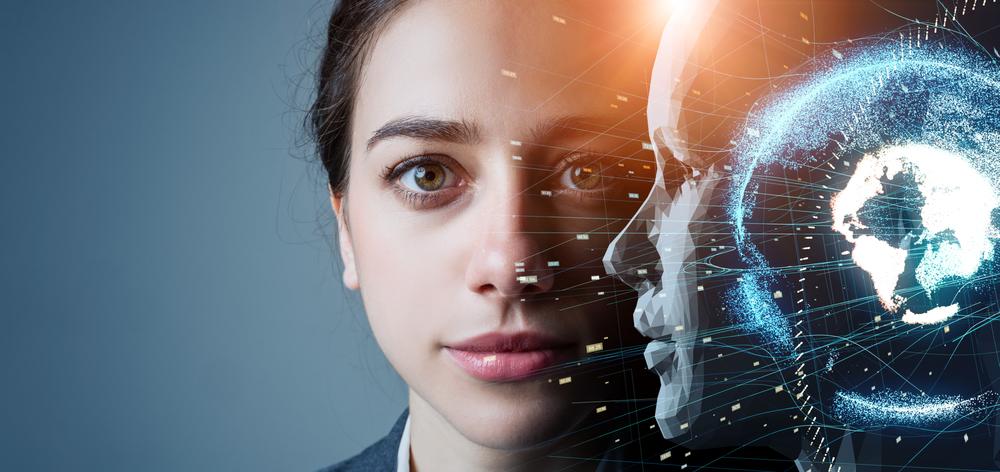
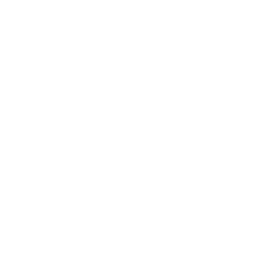
The Need for High-Quality Training Data
One can get different answers to this question, but it is widely accepted that the most important piece of the AI and machine learning process is high-quality, secure, human-annotated training data.
There are different ways to collect and structure data, with different firms having their own approach.
The problem with using unstructured or raw data is that it is very difficult to train high-quality models. In addition, the annotations can have a large impact on the performance of a model if the labels are bad quality or if the model is trained in a biased way, unbeknownst to the engineers and data scientists building it.
In order to reduce the impact of these issues, it is important to use human-in-the-loop data annotations when training a model.
Why Human-in-the-Loop?
Human-in-the-loop data annotation, or labeling, has become an increasingly important part of the machine learning process.
The use of human annotations has brought out the best in machine learning algorithms by reducing the effects of bias in the data and ensuring that models are being trained in the most effective, and most accurate, way possible.
Human-in-the-loop data annotation has several benefits, two of which are essential to the training process.
Benefit #1
Human-in-the-loop annotations are able to reduce bias in the data. In order to create a data set that is useful for training a model, it is necessary for the data set to include high-quality, diverse data, and this is not always the case.
Human-in-the-loop annotations help to reduce the chance of bias in the data that is being used, since the labelers are actively questioning and reviewing the data.
This means that the model will be able to learn from a variety of different perspectives and can be used to create more accurate predictions.
Benefit #2
A diverse set of human labelers will be able to create more accurate annotations, since they will be able to provide different perspectives on the same data. This will help reduce the chance of under-fitting the model and will help increase the overall quality of the output.
This also allows the model to be used in a wider variety of situations, increasing its applicability and building on the idea of “AI for all.”
When all people, regardless of race, age, or gender, can use AI in their daily lives, we will be able to create a more inclusive and connected world.
By having a diverse set of human labelers, we are able to increase the number of people that we can use for data annotation, and this will create a better, more inclusive model that reaches more people and ultimately solves more problems.
How DataForce Can Help
DataForce recognizes the many benefits of using the human-in-the-loop data annotation model and has a network of over one million contributors that can help diversify your AI training process.
Our large, diverse team of data annotators can help you reduce the amount of bias in your data so it is being used in the most effective way possible.
Increase the quality of the model using human-in-the-loop data annotation so it can be used in a wider variety of situations, expanding its applicability and making the solution accessible to anyone, in any setting.
DataForce offers AI solutions across multiple industries. Contact us today to learn how we can help.