Translation AI: Hype vs. Impact
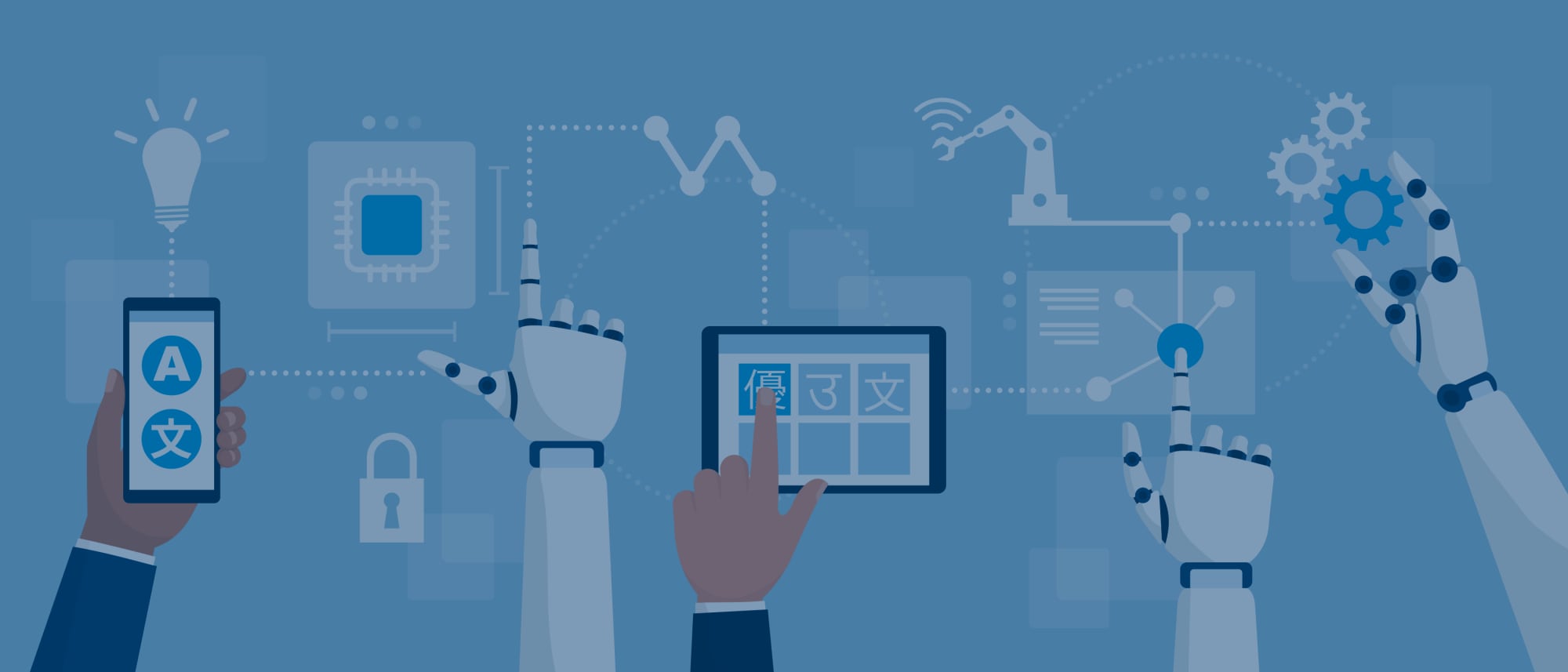
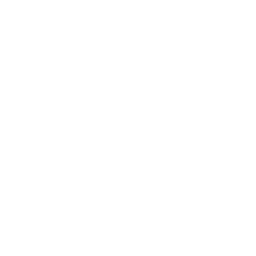
The launch of ChatGPT in late 2022 sparked immediate hype around generative AI. Many were quick to declare that AI would destroy all jobs. In the translation world, what AI is doing is enhancing productivity and opening up new opportunities.
In a recent webinar, TransPerfect’s Diego Bartolome, head of AI.NOW, and Ty Trainer, Machine Translation Implementation Manager, delved into what they call “translation AI,” a way of doing translation that stacks generative AI on top of existing technologies. They talked about how to get started implementing AI into workflows, use cases, and emerging opportunities.
Translation AI: The New Reality
TransPerfect has been doing machine translation (MT) for about 30 years. Now, we’re integrating generative AI (specifically, large language models, or LLMs) to help us do more. Translation AI is a framework for understanding this AI-integrated way of performing translation and localization. It’s thinking about AI not as a distinct, unconnected technology but as a set of technologies that work together to maximize productivity while also ensuring quality and extending savings.
How Has AI Hype Impacted Companies?
Push to implement: Companies are pressured by leadership to implement AI solutions. But they may not know how to do it, or what it will mean for operations. Ideally, it’s done slowly and thoughtfully in a way that considers different use cases and requirements for various business units within the company.
IT solution to “the language problem”: Translation has shifted from being owned by marketing to being owned by IT departments. Companies think human translation is expensive, and they see generative AI as the most efficient solution. But often, the people involved in the content and localization processes are not consulted before solutions are implemented. To be effective, companies need to drive change with people, as technology alone will not solve everything.
Reshaping content and localization goals: The translation industry is becoming more technological, which is reshaping roles and challenging traditional processes—even creating new career paths. People need to understand the technology and how best to incorporate it into workflows.
The AI Maturity Model
There is a temptation to implement AI for the sake of it, but this is the wrong approach.
Don’t implement AI because it’s there; do it to solve a business problem. Many companies are in the early stages of implementing AI, and the best way for them to “move up” is through experimentation. We experiment with customers on their AI journey. We create pilots and minimally viable products (MVPs)—the first version of a product or service that allows customers to understand when generative AI or AI can help the business. If it works, we scale. If not, we try something else.
From Hype to Reality: Where Are We Seeing Generative AI Applied Most Usefully?
We look at how LLMs can combine with existing translation workflows to improve processes. We ask: How can we stack—or more meaningfully incorporate—generative AI into translation-specific workflows? We can use generative AI in conjunction with existing AI technologies that companies are using for translation, i.e., neural machine translation (NMT).
Machine translation is a mature application of AI. It’s been at the peak of productivity for 15–20 years. LLMs are a more nascent technology. While NMT is designed for translation, LLMs are open-ended in what they can do. At TransPerfect, we have found in almost every use case that NMT outperforms generative AI in terms of translation quality. We leverage both for their respective strengths.
Here are three areas where we are seeing success:
- Accelerating post-editing tasks
- Maximizing translator efficiency
- Offering greater translation fluency
Once we have our source content, we use AI to optimize it. This is a fully automated step. We then use translation AI (NMT + LLMs) to translate and perform post-editing, with a human-in-the-loop option.
Emerging Opportunities
Synthesis AI is being used to draw insights from content. We foresee being able to incorporate synth AI for multilingual content generation. Instead of producing the content and then translating it into multiple languages, we’d be producing it in multiple languages. Eventually, instead of translators, we’d have in-language reviewers.
This would happen first with lower-risk content. For example, you could feed synth AI a prototype, a few features, and an image to write multilingual product descriptions for different retailers.
Assess Translation Needs
Do you need machine translation for projects that would be too expensive if you used humans only? Do you need real-time translation to quickly reply to a large number of customers in various geographies? Do you want to increase the efficiencies of your human translation process?
Look at samples of documents that need translating. How many and which languages do you need? What are your quality requirements? Do you have historical translations to draw from? We can use what you have to calculate the approximate level of productivity and quality you can expect to see from MT.
The best thing companies can do to integrate AI is to start small. An organization doing human-only translation can’t expect to jump immediately to a fully automated workflow.
And remember that data is critical for both AI model training and for proving ROI. Organizations need to reliably report on ROI. Having a reliable way to use data to measure impact is crucial. Finally, integration doesn’t happen overnight. Expect to build, learn, and iterate.