Streamlining Dispute Resolution through AI and Advanced Analytics
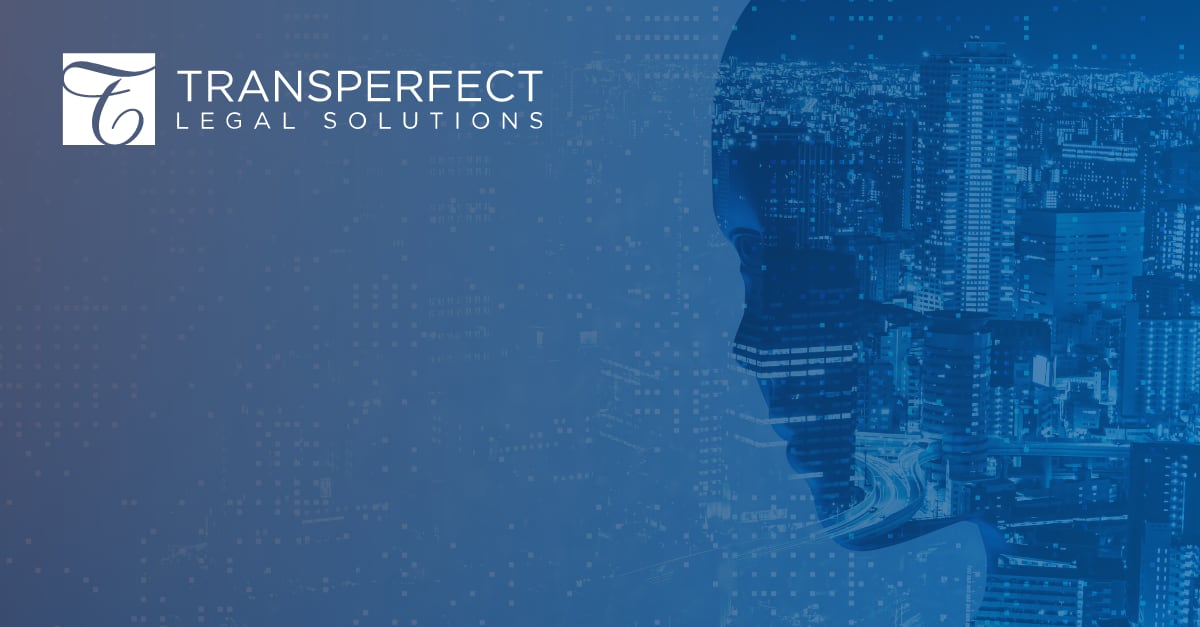
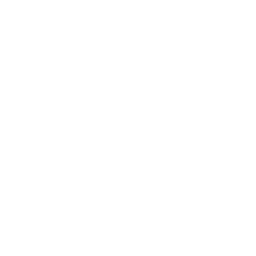
Big Data Background
While the use of artificial intelligence (AI) and advanced analytics in e-discovery can often seem like a black box, our webinar on Advanced Analytics & AI in Complex Litigations & Investigations from January 2023 aimed to break down the various technologies and demonstrate their value at all stages of a dispute.
Panelists argued that it is important, and in many cases necessary, to utilize AI and advanced analytics due to the sheer volume and diversity of data involved in present-day litigations and investigations.
As TLS President of Consulting & Information Governance Daniel Meyers stated, “The haystack is larger than it has ever been before, but this does not mean there are more needles; it just means that you have to work harder to find the needles.”
This is where deploying advanced analytics and AI is essential to separate the digital wheat from the chaff.
Pre-Review Analytics/AI
Document review is the most expensive part of the e-discovery process, typically comprising 73 cents of every dollar spent on discovery. Accordingly, the only way to truly control e-discovery budgets is through a laser-sharp focus on filtering out the digital debris before promoting documents to a document review platform. Moving beyond traditional filtering approaches (search terms, date filters, and deduplication), the panelists explained that an essential step to addressing large datasets is deploying Pre-Review Analytics—leveraging analytics and AI to further identify and isolate non-responsive information prior to commencing document review.
There are several tools to identify data that can be excluded from hosting and review. One important tool is email domain filtering—identifying emails that contain search term hits but, in reality, are spam, industry newsletters, or other automated emails without meaningful human content. Concept clustering is another extremely useful tool, as it organizes documents based on the presence of similar terms or concepts and can quickly identify large groups of documents about trivial and/or non-relevant topics that happen to hit on search terms. Concept clustering can likewise help to refine search terms and identify pockets of data that warrant closer analysis.
TLS Vice President of Consulting Services Michael Kriegal summarized, “The idea is that you are getting top-level information about your data without looking at each individual document.”
Panelists cited a case study about a global manufacturer facing a class action over alleged product defects. While more than 80 percent of the documents were culled after deNISTing, keyword searches, and date filtering, an additional 1.4 million documents were filtered out using Pre-Review Analytics. This resulted in cost savings of over $1 million by eliminating an additional 1.4 million documents from the document review universe.
Investigative Analytics/AI
In addition to using analytics to further cull the dataset, clustering can be especially helpful in internal investigations by quickly identifying whether there is any truth to the allegation(s) under examination. Another investigative tool is often referred to as “find more like these.” If a document is identified as being of interest, the system can find similar documents based on the words and phrases in the document. Communication mapping is another visual tool that shows who is talking to whom and how much. For example, communication mapping could show that an employee is consistently sending emails to their personal email address—raising a red flag.
Review Analytics/AI
Once the team is at the review stage of the project, there are additional tools that can be used to streamline review and further reduce costs. First, email threading is used to group emails (replies, forwards, etc.) together. This can reduce the review population by suppressing lesser-inclusive emails fully contained in later-in-time emails in the thread. Email threading generally improves coding consistency, since emails in the same chain are reviewed and coded together. Second, one of the most powerful tools in a large review is technology-assisted review (TAR).
While there are several different TAR flavors and workflows, TAR, or “predictive coding,” at its core “is a process where a smaller set of documents is reviewed and coded by subject matter experts, and those [coding] decisions are used to create a model that can extrapolate the coding decisions on the remaining unreviewed document set,” said TLS Director Brittany Field. Several factors—including data types, volume, rate of responsiveness, and timeframe—will determine the most appropriate workflow. A TAR 1.0 model is ideal for large datasets when time and resources are especially tight. A TAR 2.0 workflow is best for low-richness datasets or where the lawyers want to ensure human eyes are on every document that is part of the production.
Lastly, it is important to consider whether foreign languages are in the dataset and how best to handle those, and whether enhanced machine translation or human translation will be required. While many languages are supported by the various TAR platforms, there may be a document translation requirement for production.